A key goal of modern medicine is "precision medicine", the purpose of which is to personalise treatment based on the patient’s specific characteristics of the disease. Radiomics is rapidly emerging as a personalised medicine technology and is currently one of the most interesting fields of research.
To understand what Radiomics is, it is necessary to start by saying that some tumours are characterised by molecular alterations, such as genomic alterations. Given that it is possible to define these alterations, it is generally necessary to have a sample of the neoplastic tissue, which is obtained by biopsies or invasive surgical interventions. Currently, however, imaging diagnostics can enable tissues to be characterised in a non-invasive manner and, in some cases, can enable the profound phenotypic differences to be visualised. Since tumours are heterogeneous in their volume and change over time, diagnostic images can provide a full view of the entire tumour and can be repeated over time to monitor the changes induced by therapies.
Through Radiomics, the medical images, obtained by CT, MRI or PET scans, are converted into numerical data. They are calculated by calculation tools and their analysis often required the use of advanced techniques, such as artificial intelligence methods.
This huge wealth of numerical data, which could not possibly be processed by means of simple visual observation, defines many characteristics of the tumour and the surrounding environment, related, for example, to its shape, volume and tissue structure.
It is possible to study the relationship between the data obtained from the images and the molecular and genomic characteristics of the tumour, with the final aim of extracting indications - directly from the images - regarding the aggressiveness of the disease, the most indicated therapies and its response to treatment.
Hopefully, in the near future, radiological imaging and radiomic models will be used as a decision-making support to clinical practice, to improve diagnostic accuracy and prognostic power.
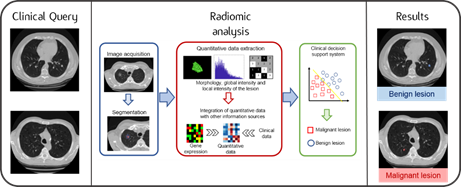
Graphical abstract of the Radiomics workflow: quantitative parameters calculated from clinical images are analysed in combination with the patient’s biological, genetic and clinical characteristics. Thanks to the use of advanced techniques, it is possible to obtain useful information for diagnosis and for personalising treatment.